Program Day 5 - January 13, 2023
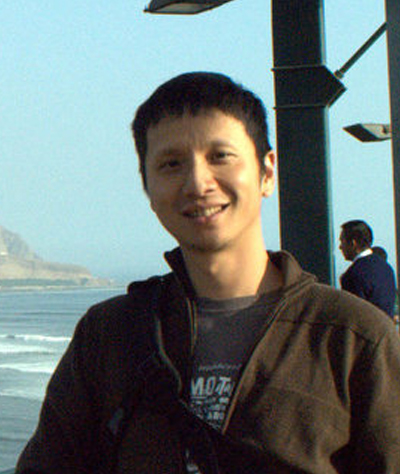
Tianming Yang
9:00 - 10:00 :- Evidence transformation and accumulation in the parietal cortex- Evidence transformation and accumulation in the parietal cortex
Bio: Dr. YANG Tianming obtained his B.S. degree in the Department of Biochemistry at Fudan University. He received his Ph. D. in neuroscience at the Baylor College of Medicine, Houston, Texas, investigating the neural plasticity in visual cortices under the advice of Dr. John Maunsell. He had his potsdoc training at HHMI and University of Washington with Michael Shadlen. Since 2013, Dr. YANG works at the Institute of Neuroscience as Investigator and Head of the Laboratory of Neural Mechanisms of Decision Making and Cognition.
Title: Evidence transformation and accumulation in the parietal cortex
Abstract: The brain makes decisions by accumulating evidence, which comes from the sensory system. It is not well studied how sensory information is transformed into accumulable evidence in the brain. We trained macaque monkeys to perform a two-stage probabilistic reasoning task in which the evidence for accumulation had to be first assembled from sensory signals. We show that posterior parietal cortex neural activity reflected the transformation from sensory inputs to accumulable evidence and the accumulation of evidence for decision making. The presence of both computational stages indicates that evidence accumulation signal in the parietal cortex is computed locally.
Session Chair: Arjun Ramakrishnan
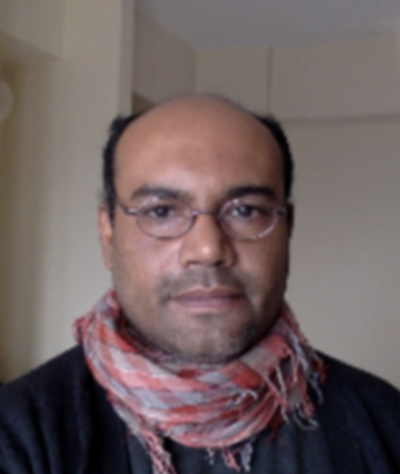
Dipanjan Roy
10:00 - 11:00 :- Electrophysiological and neuroimaging evidence of how the brain handles salient distraction, surprise, and uncertainty
Bio: Dipanjan Roy’s Cognitive Brain Dynamics Lab studies neurodynamics of human memory, perception, attention, and emotion processing during typical and atypical brain development using behavior, EEG, MEG, and fMRI. His group has made seminal contributions in multiscale computational modeling, Age-associated alteration in neural communication and synchronization between brain areas, Multisensory speech processing and role of attention, Brain connectivity and neural flexibility in Autism, Emotion and perception dynamics in Aging and neurodevelopmental disorders. His lab is currently located at IIT Jodhpur Center for Brain Science & Applications under the School of Artificial Intelligence & Data Science and the National Brain Research Center Manesar Haryana.
Title: Electrophysiological and neuroimaging evidence of how the brain handles salient distraction, surprise, and uncertainty
Abstract: What fundamental property of our environment would be most valuable and optimal in characterizing the perceptual and emotional dynamics we experience in daily life? Empirical work has shown that an accurate uncertainty estimation is necessary for optimal perception, learning, and decision-making. However, the role of this uncertainty in governing our affective dynamics remains unexplored. Using Bayesian encoding, decoding, and computational modeling on a large-scale neuroimaging and behavioral data on a passive movie-watching task, we showed that emotions naturally arise due to ongoing uncertainty estimations about future outcomes in a hierarchical neural architecture. Several prefrontal subregions hierarchically encoded a lower-dimensional signal highly correlated with the evolving uncertainty.
Session Chair: Arjun Ramakrishnan
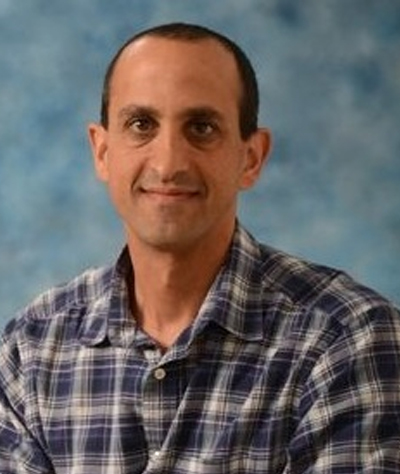
Dino Levy
11:30 - 12:30 :- Using EEG neural signals for predicting future choices and population marketing success.
Bio: Prof. Levy is an associate professor and the head of the Neuroeconomics and Neuromarketing lab at the Marketing Department at Coller School of Management since fall of 2012. He is also the head of the undergraduate program in management at the Coller School of Management. Prof. Levy has a BA in Economics from Tel-Aviv University and an MA in Psychobiology, in which he graduates Magna Cum Laude, also from Tel-Aviv University. He received his PhD in 2008 from the Neurobiology Department at the Weizmann Institute of Science in the field of animal models of drug addiction. He then continued for post-doctoral studies at the Center for Neuroeconomics at NYU in which he examined the neural value representations of both primary and monetary rewards. In his lab they use an interdisciplinary approach, which involves quantitative economic theories, combined with advanced behavioral methods and theoretical models from psychology, marketing, and economics with neuroscience techniques such as functional magnetic resonance imaging (fMRI), Electroencephalography (EEG), and eye-tracking. The main aim in their studies is to try and better understand how we make decisions and what are the neural mechanisms underlying value-based choices. The projects in the lab range from examining the neural correlates of value computations and the common currency network, deciphering the neural mechanisms of irrational choice behaviors both in humans and in C.elegans, through looking for a common denominator between basic visual perception and value computations, to projects that aim to predict future preferences and population success of marketing stimuli using neural signals.
Title: Using EEG neural signals for predicting future choices and population marketing success
Abstract: A basic aim of marketing research is to predict consumers’ preferences and the success of marketing campaigns in the general population. However, traditional behavioral measurements have various limitations, calling for novel measurements to improve predictive power. Hence, there is increasing demand within consumer-neuroscience (or neuromarketing) for objective neural measures to quantify consumers’ preferences and predict responses to marketing campaigns within individuals and the general population. In my talk, I will present several studies where we use neural signals measured with electroencephalography (EEG) in order to do so. In the first study, subjects viewed individual consumer products in isolation, without making any actual choices, while their neural activity was measured with EEG. At the end of the experiment, subjects were offered choices between pairs of the same products. Using recent techniques for relating neural measurements to choice prediction, we demonstrate that these measures predict subjects’ future choices. In the second study, we recorded the EEG signals of subjects, as they watched commercials of food products. We introduce a novel approach in which instead of using one type of EEG measure, we combine several measures, and use state-of-the-art machine learning algorithms to predict subjects’ individual future preferences over the products and the commercials’ population success, as measured by their YouTube metrics. Importantly, we demonstrate, that for all of our predictions, the EEG measurements increased the prediction power of the questionnaires. In the third study, we aim to develop a novel neuropredictive tool based on deep learning convolutional networks for analyzing the EEG signal which can be used to estimate subjects’ valuations and future choices. Overall, our analyses methods and results show great promise for utilizing EEG measures by managers, marketing practitioners, and researchers, as a valuable tool for predicting subjects’ preferences and marketing campaigns’ success.
Session Chair: Arjun Ramakrishnan
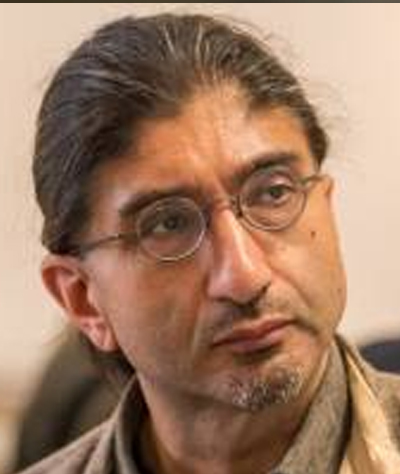
Maneesh Sahani
14:00 - 15:30 :- The dynamics of robustness in motor cortical circuits.
Bio: Maneesh Sahani is Professor of Theoretical Neuroscience and Machine Learning at the Gatsby Computational Neuroscience Unit at University College London (UCL). Graduating with a B.S. in physics from Caltech, he stayed to earn his Ph.D. in the Computation and Neural Systems program, supervised by Richard Andersen and John Hopfield. After periods of postdoctoral work at the Gatsby Unit and the University of California, San Francisco, he returned to the faculty at Gatsby in 2004 and was elected to a personal chair at UCL in 2013. His work spans the interface of the fields of machine learning and neuroscience, with particular emphasis on the types of computation achieved within the sensory and motor cortical systems. He has helped to pioneer analytic methods which seek to characterize and visualize the dynamical computational processes that underlie the measured joint activity of populations of neurons. He has also worked on the link between the statistics of the environment and neural computation, machine-learning based signal processing, and neural implementations of Bayesian and approximate inference.
Title: The dynamics of robustness in motor cortical circuits.
Abstract: The rich repertoire of skilled mammalian behavior is the product of neural circuits that generate robust and flexible patterns of activity distributed across populations of neurons. Although decades of associative studies have linked many behaviors to specific patterns of population activity, association alone cannot reveal the dynamical mechanisms that shape those patterns. Here, we consider the dynamics of primate motor cortex perturbed by optogenetic and electrical microstimulation during reaching behavior. We develop a novel analytic approach that relates measured activity to theoretically tractable, dynamical models of excitatory and inhibitory neurons. This computational model captures the dynamical effects of these perturbations and demonstrates that motor cortical activity during reaching is shaped by a self-contained, low-dimensional dynamical system, acting within a subspace that is oriented so as to be robust to strong non-normal amplification within cortical circuits. The results resolve long-standing questions about the dynamical structure of cortical activity associated with movement, and illuminate the dynamical perturbation experiments needed to understand how neural circuits throughout the brain generate complex behavior.
Session Chair: Sridharan Devarajan
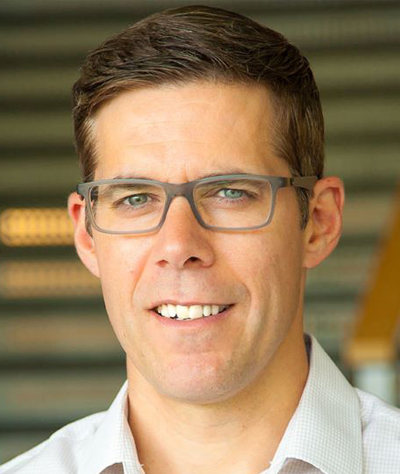
John Seymour
16:00 - 17:00 :- New challenges and opportunities in implantable electrode arrays
Bio: Professor Seymour’s research is in the area of advanced neurotechnology with a focus on translational brain-machine interfaces. His research has addressed topics including reduced tissue reactivity and improved optical, electrical, and mechanical characteristics of bioelectronics devices. He is an Associate Professor in the Department of Neurosurgery at the McGovern Medical School at the University of Texas Health Science Center at Houston. He also holds an appointment as Adjunct Assistant Professor in ECE at Rice University. He was recently awarded the University of Texas Rising Stars Award. He earned his B.S. with Honors in Engineering Physics from Ohio State University and his M.S. and Ph.D. in Biomedical Engineering from the University of Michigan. His industry experience includes working at NeuroNexus as a Principal Scientist. Dr. Seymour’s other academic experience includes serving as research faculty in the Department of Electrical Engineering at the University of Michigan where he developed novel neural interface systems including neuroscience mapping tools.
Title: New challenges and opportunities in implantable electrode arrays
Abstract: The future story of minimally invasive implantable neuroelectronics has yet to be written. The application demands advanced manufacturing, miniaturized electronics, and a revolutionary approach to packaging. As challenging as those problems are, even more difficult is defining the best electrode and substrate geometry to optimally record from multiple brain regions. This talk will focus on the process of choosing the electrode/substrate geometry, trajectory, and target with input from lead field theory and biophysical models. These efforts have driven us toward novel depth arrays in the applications of BCI and seizure localization but apply to any electrode recording or stimulation application. Finally, we will share our progress in manufacturing to achieve our goal of large-scale depth arrays.
Session Chair: Arjun Ramakrishnan